Machine Translation – Mikel Forcada
Interview with the President of EAMT
Bronwyn Hogan, VTQ
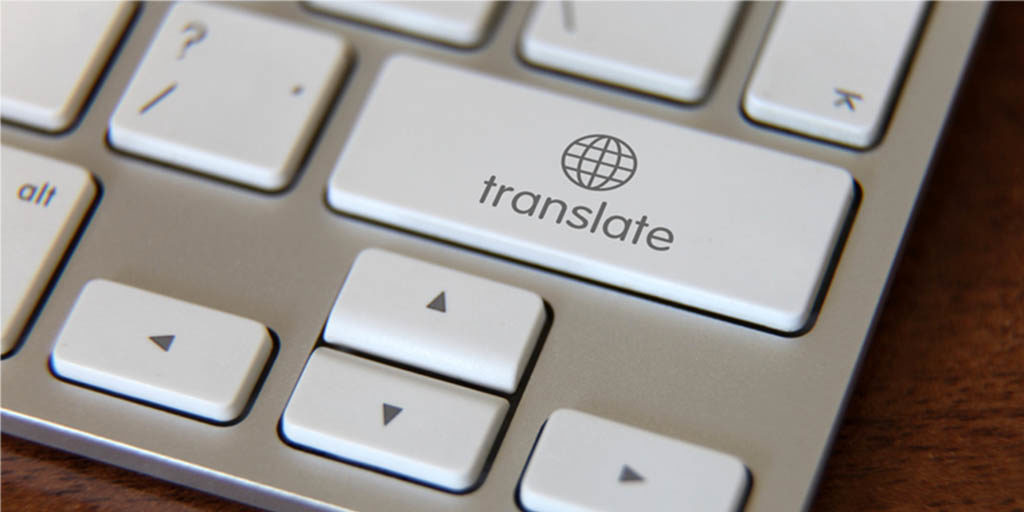
Mikel Forcada is the President of the European Association for Machine Translation. Professor at the Universitat d’Alacant and Founding Partner and Chief Research Officer at Prompsit Language Engineering. VTQ caught up with Mikel Forcada to discuss the current landscape of Machine Translation.
What is Machine Translation (MT) and why are more and more companies considering it as part of their overall solution?
MT is an automated process that translates source text into target text. The result is usually very different from a professional translation, and therefore cannot be normally used for the same purpose. Sometimes it is not that different and can, therefore, be postedited (ideally by properly trained professional translators) to produce, in an economically advantageous way, target text which is as adequate as target text that has been professionally translated without using MT.
Companies are adopting MT because it may drastically increase translation productivity (that is, number of words translated per day by a translator) and therefore lead to reduction in costs or shorter marketing cycles.
What kind of companies have adopted machine translation?
On the one hand, companies that have to manage multilingual content and may adopt MT as part of their content translation process. MT would be used by in-house professional translators or localizers who would postedit MT output or interact with it in some way or would be sent to external posteditors.
On the other hand, LSPs, that is, translation providers, have adopted MT to increase their throughput and reduce costs, making them more competitive.
How much progress have you seen in recent years in the field of machine translation?
I started doing MT around 1999, but I was studying it closely since 1997 or so. In these twenty years, I have seen statistical machine translation (which was already quite established as a research topic at the turn of the century) displace rule-based MT for many language pairs and applications. This also meant putting parallel corpora — that is, the work of professional translators — in the spotlight. Now neural machine translation, which also leverages on the work of translators, has started to displace statistical machine translation, particularly for those language pairs and applications where a lot of parallel text is available. So yes, I have seen at least two radical changes in the way MT is done.
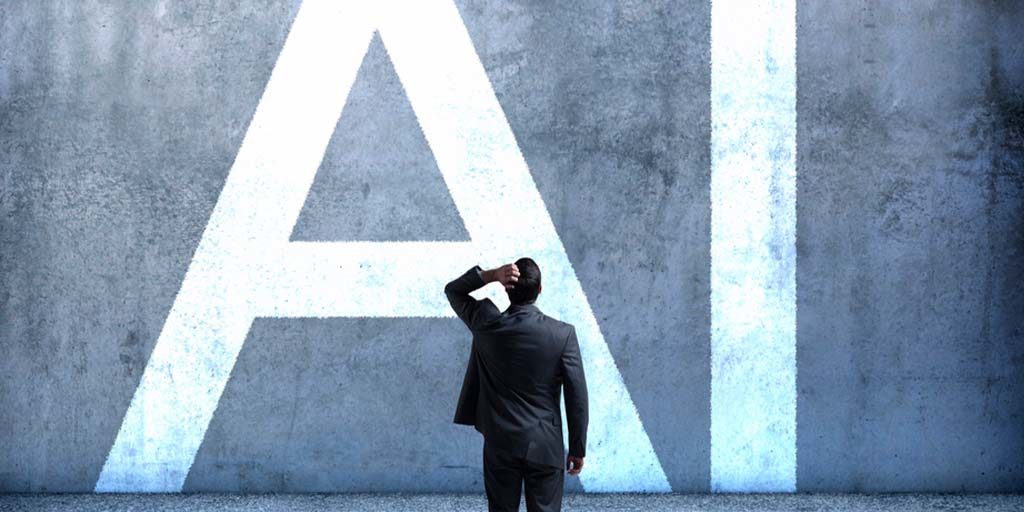
What challenges and drawbacks do you see in adopting machine translation at present? Do you see this changing over the next three to five years?
Small languages and specialized domains may not have enough parallel text available to train a statistical or neural machine translation system that produced usable (that is, post-editable) output.
There are still many aspects of MT evaluation which are not clear. Progress in MT as a field is driven by automatic evaluation metrics (such as BLEU) that compare how close MT output is to one, and if you are very fortunate, a couple of reference translations. However, the ability of such automatic evaluation measures to predict post-editing effort or the usefulness of raw MT output “as is” is still quite limited. This means that MT, as a research field, may sometimes be going around in circles when it thinks it is progressing. Perhaps “a statistically significant increase of 1 BLEU point” does not mean that much in the translator’s workstation.
Statistical MT to some extent, and neural MT more clearly, tend to produce very fluent output which may however not be a proper translation of the source: words may be missing, or unnecessary words may be added. This was not the case with good old rule-based MT: its “mechanical” output did not miss or delete words so much, and errors would fly in the face of posteditors. Now posteditors have to be very careful!
In your opinion, as Chief Research Officer, would you consider machine translation to still be in its infancy?
I think machine translation is a mature field. For large language pairs and domains where enough parallel text is available, it is often the case that MT is a key component of real-world, competitive translation processes. Life is harder for other languages and domains though. But definitely not in its infancy!
As machine translation develops and technology improves, do you see the current challenges being viewed as minor issues when we look back in a few years?
I find it hard to say. I believe any technology that automatically produces content that has to be used or acted upon by a human user in one or another way will always face challenges as people vary along many dimensions: language abilities, cultural background, capacities. I think these basic challenges will remain, and new challenges will appear as machine-generated output will also enter the culture of people. The mutual interaction between machine translation and its users will surely pose many interesting questions.
Can you explain the difference between Machine Translation and Neural Machine Translation?
When you say “machine translation”, I will assume you are referring to statistical MT.
Statistical MT uses large “phrase tables” storing “phrase pairs”, each containing a contiguous stretch of source language words (“source phrase”), the corresponding contiguous stretch of target language words (“target phrase”), and some statistically derived scores for that pair. These phrase pairs are extracted from parallel texts during training; they are found there. Then, when a new source segment comes up for translation, it is dismantled in contiguous stretches in all possible ways, and they are looked up in the phrase table. Then, for each possible “dismantling”, target phrases are stitched together (with reordering if necessary) in all possible ways. Scores are used to select the best possible “collage”.
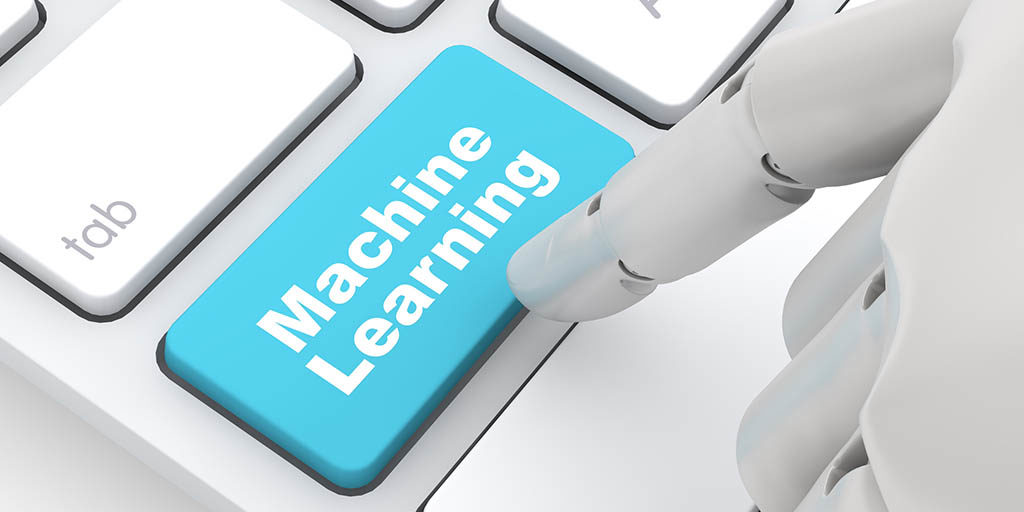
Neural machine translation is different. It is called neural because it is performed by networks of small computational units that roughly resemble brain neurons. The strengths of the connections between these units are selected so that they interact and as a result behave as desired. The source sentence is read, word by word, by a neural network called a decoder and a vector representation (that is, an array of numbers representing the activation levels of its neurons) is built for the sentence. Then another neural network called a decoder, predicts the most likely target words one by one by looking at the representation of the source sentence.
The output is not a collage, but the product of a series of word predictions informed by the source sentence.
Do you see normal machine translation as a dominating technology in the localization industry in the coming years and what technologies do you also see on the horizon and what comes after Neural Machine Translation?
I am not a visionary and I might be very wrong, but I’ll give it a try. I don’t know what comes after neural machine translation, but one piece that seems to be missing is user modelling. If MT output is going to be postedited, the MT system should be able to monitor and model the professionals that are postediting it, so that its output suits them better.
Current MT systems are still far from getting in their users’ shoes. Modelling users will surely need the assistance of artificial intelligence of some kind, deep-learned neural or other. Related to this is quality estimation, a hot but very hard field of MT research, challenged by the enormous variability among translators. If a translator has more than one technology available, it would be good if decisions could be made on their behalf as to which technology is the best for the current segment or passage. This means that the quality, that is, the usefulness, the ability to reduce translator effort, needs to be accurately estimated for each possible technology. Having a translation technology broker which tells translators where to invest their effort obviously implies modelling each individual translator.
In relation to bots and machine-to-machine conversations, how does this impact content and growth in the coming years?
I am not familiar with bots or machine-to-machine conversations. What I see is that human users consume more and more machine-generated content (and are learning to use it and react to it), and that some of this content may be ingested back into machine translation systems, with a range of consequences. Clearly, computational agents may learn to deal with and react to the language of content produced by other computational agents, dealing to interesting interactions, and perhaps to a new kind of content being exposed to human use. The 2018 edition of the EAMT Best Thesis Award went to Daniel Emilio Beck’s thesis “Gaussian Processes for Text Regression” [I have not read the thesis completely, but I know some of Daniel’s work]. Text Regression is the task of modelling and predicting numerical indicators or response variables from textual data.
How does Text Regression relate to Machine Translation?
About half of Daniel Beck’s thesis used text regression techniques to estimate the quality of MT; in particular, to predict post-editing time.
How do you see the progression in Text Regression changing Machine Translation?
Predicting post-editing time is a very hard task, particularly as there is a lot of variability in the professionals that post-edit MT. Work by Daniel’s advisor, Prof. Lucia Specia, and Dr. Trevor Cohn, used text regression techniques to model individual posteditors. But what happens if a new post-editor comes in? Text-regression techniques could be applied to their post-edited output to model them and get more realistic estimates of post-editing time.
This article first appeared in VTQ Magazine.
Read more: https://vtqglobal.com/