The MT Tipping Point - Featuring Konstantin Savenkov
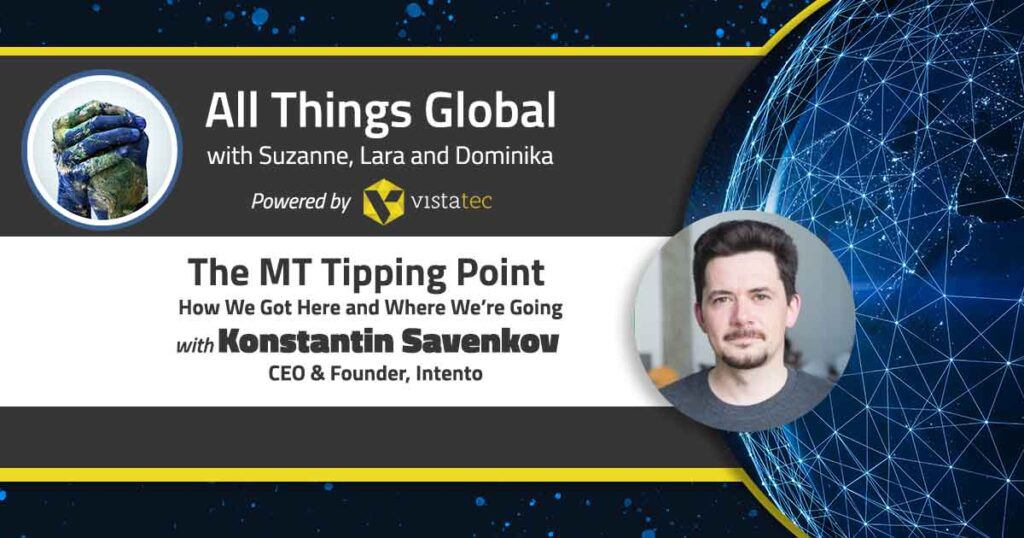
Episode 4 of Vistatec’s All Things Global went live on June 3rd, 2021, with guest Konstantin Savenkov and hosts Suzanne Marie Frank, Lara Daly, and Dominika D’Agostino. Konstantin is the founder and CEO of Intento, spending time with other types of companies throughout his career. Intento is an “abstraction layer for Cognitive Services, with multiple Artificial Intelligence (AI) vendors via a single API integration and up to 23x performance/price efficiency from choosing the right model for your specific use.”
Konstantin shares his journey—how he has gotten to where he is today with Intento. Konstantin explains that he spent some time teaching at University and working toward his Ph.D., then left to write recommender systems for books and music. During that time, he notes that the exciting part was that they built a couple of startups that seriously disrupted the content publishing market for music and books. The switch from buying files and CDs to listening to music via streaming services allowed the launch of a music streaming service alongside Spotify in Eastern Europe.
Konstantin notes how exciting it is to see how new technology goes through different stages of adoption, “disrupting massive fragmented market with long supply chains.” Konstantin and his partner decided to launch Intento in early 2016. The idea was to help companies work with AI in general since large enterprises often fail when first attempting to use AI. Those failures are not because the model is inaccurate; instead, people approach working with AI solutions wrongly. People tend to think it’s the same software as their Excel spreadsheet software—simply find a vendor, have a pilot, and scale it up.
Most of the AI problems involve translation and localization. Almost every large company in practically every department needs translation and machine translation (MT), even if they don’t realize they need it. People have been attempting to work with machine translations since 1950 – while we think it’s very modern, it’s one of the oldest areas involving people working alongside technology. People must learn to be more proactive with AI in a broader range of fields. As an example, think about desktop publishing or anything related to speech and video.
Konstantin notes specialist tools are necessary; Intento spent a couple of years looking for the right solution, combining the suitable models, and doing proper automated post-editing to make them more suitable for a specific use case. Then you get one set of models and use them to control across the whole enterprise—not only localization departments but also touching the day-to-day lives of virtually every employee in the company. This increases productivity when working with multilingual content. Intento found a place that had a need that was in the localization space.
Lara Daly, Vice President in Expanding Global Community for Vistatec, has questions for Konstantin, delving deeper into the fact that he worked in streaming music and publishing before translating. Konstantin says he was an early employee in a couple of startups. However, translation is the next place to help maximize the benefits of AI. Lara asks what Konstantin sees right now when looking at localization compared to what he saw when he first started—the trajectory for deploying AI and MT in localization?
Konstantin says that for him, from a business standpoint, it feels very similar. Konstantin discovered a complex ecosystem with technology vendors, AI vendors, data owners, service providers, and software vendors. What became clear was that when new technology is applied to existing large markets, it goes through certain stages of adoption. It must first be shown that new technology can deliver better results than old technology. Then translators and end-users must learn to work with machine translation.
If you look at the extended supply chain, every step adds value, so if you just bring something to end-users that creates lots of value, then the question is, how will this value be redistributed along the supply chain? Businesses are at the point where they are playing catch up as the process evolves, adopting different tools to incorporate MT in daily works. First, translators must understand how machines work; then, native speakers will review machine reports, improving the machine translation models. Through this process, the translators change from being “support engines” to people involved in localization; they train the technology.
Audience member Nicole Kittle, Roku asks Konstantin how expensive it is to train up like this and its value. She says that ideally, you want a trained engine to work on your content, but if you have technical content, is there any value to an untrained engine, noting that their people are still very hesitant with machine translation, particularly in life sciences. Konstantin explains that you cannot just blindly use a stock engine, and you cannot throw your content into any customizable system then take it and use it. You have to know how it works on your particular content before you go all-in into production. While they do use stock engines in some cases, they add some things on top to make them more appropriate.
When editing and evaluating performance, you may see specific issues with terminology, which must then be “trained.” With any language, there must be consistency in the translation, i.e., formal or informal. There is a question regarding ways to estimate the quality that comes out of the engines. Konstantin says that quality summation is a broad and complex topic and that everyone is looking for the “holy grail.” He notes that they played with many different technologies from vendors and that most of the translation will be under the bell curve—not too good or too bad.
Audience member Jeff McIntosh, Fedex has a question regarding the industry average on the amount of engine training or how often the engine training should occur. Konstantin answers that it would likely depend on the volume of new data; rarely does a company have enough data to update engines more than quarterly; the data must be cleaned, and cleaning typically filters 60-80 percent, not necessarily because of bad translations, but because it’s not appropriate for training. He notes that it is a factor with the budget, obviously, but that at least once a year, they update to see whether they are on par with the industry average. If data sets increase about ten percent, you will get a boost from an update, less than ten percent; you probably shouldn’t expect much.
Rachel Hegeman, Pitney Bowes wonders how much difference there is in the MT quality that comes out when content is specifically written to optimize MT. Konstantin says this is a critical topic; when they evaluate machine translation engines, they can pinpoint source sentences that create problems for the engines—you can see where most of the issues come from, resulting in essential feedback.
There are sentences that create issues for all engines in specific domains, and they can be addressed by writing differently. Konstantin says they previously published guidelines on how to write for machine translation based on their observations. He further notes that he uses a unique way of writing emails in English to avoid any ambiguities since there can be issues with colloquialisms or even typos.
Viktor Pless, LogMeIn asks if an engine has been trained with custom data from their own material, would they be better off using a stock engine that has been very fine-tuned? Konstantin states in some instances, you can rely on a glossary to capture your endowment terminology but have to train, particularly for new terminology that is very specific to your industry. There must be sufficient context, and if there’s not, the engine must be customized.
The final questions are regarding the tone of voice. How can it be fixed when MT is deployed for multilingual content—addressing the tone of voice beforehand? Konstantin addresses gender bias in some instances, noting it can be a lack of context. Even if you know your tone of voice, the MT engine is mostly just blindly guessing if you have a short sentence with little context. In addition, the tone of voice can be a problem when translating from English because you may get a mix of formal and informal.
About Vistatec
We have been helping some of the world’s most iconic brands to optimize their global commercial potential since 1997. Vistatec is one of the world’s leading global content solutions providers. HQ in Dublin, Ireland, with offices in Mountain View, California, USA.