
In this interview, Monika discusses the relationship between translation quality and trust, new roles for translators, and innovating with language quality models in the age of AI.
Translation Quality
in the Age of AI
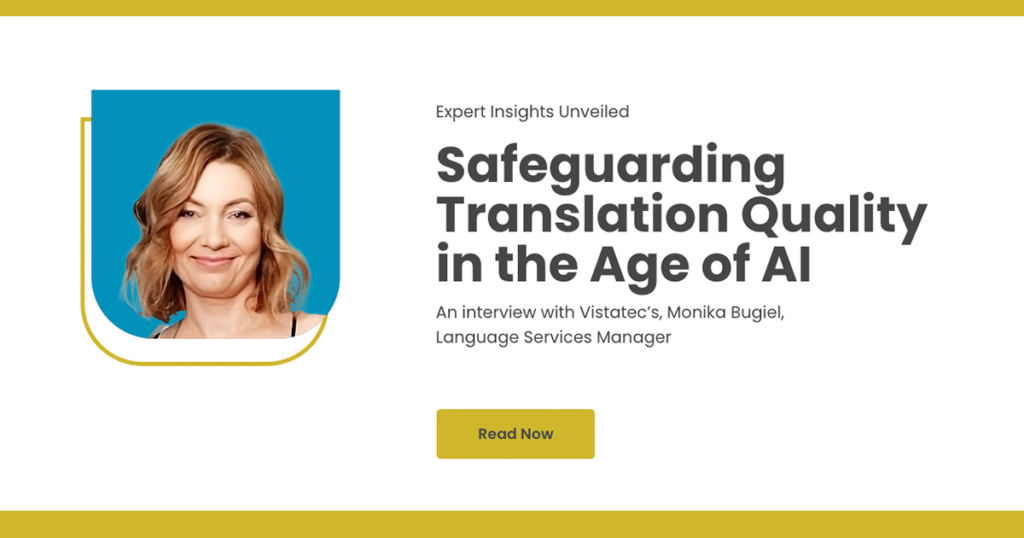
Subscribe to this content series and be the first to be notified when new content is released
Subscribe to this content series and be the first to be notified when new content is released
Safeguarding Translation Quality in the Age of AI
An interview with Vistatec’s Monika Bugiel, Language Services Manager
AI-powered translation is now everywhere. Google Translate. Bing Translator. ChatGPT. Global businesses are keen to get started so they can deliver content quickly and at scale across the globe to their multilingual customers. However, alongside the excitement for this new technology is the knowledge that the quality may not yet be good enough for many purposes. AI translations can contain bias, errors, and ‘hallucinations,’ and while brands may be in a hurry to capitalize on productivity and cost savings, poor quality is something most can’t afford. As the demand for translation has never been higher, these quality challenges must be resolved quickly. This is where the language services industry is stepping up with gusto: developing tools, processes, and approaches to ensure the quality of AI translations at speed and scale.
To learn more about how LSPs are innovating on quality processes in this age of AI, I met with Monika Bugiel, Vistatec Language Services Manager. She is a 23-year veteran of the localization industry and an expert in translation quality assurance. In her role at Vistatec, Monika focuses on strategy and processes related to quality assurance and quality control and manages a team of language quality specialists. We spoke about how translation quality impacts trust in a brand, mitigating risk with AI translation models, and what needs to happen to drive human-level quality.
A customer has more trust in a company when their content is high quality, free of errors, and well-written with good structure, logic, and flow. It’s well known that machine and AI translations do not yet reach human quality levels, yet businesses are rushing to use AI for cost and speed gains.
Monika: Brands are putting themselves at risk if they just rush headlong into AI translation. It takes a lot of time and effort to build a reputation, but not much time to destroy it. No enterprise that wants to come across as a reliable company would want mistakes in its highly visible content.
Content translated by AI without human intervention is rarely entirely fit for most business purposes. AI is trained on massive amounts of broadly available data, which allows it to acquire language knowledge and patterns from diverse sources. That data can be full of errors, bias, or misinformation. You can’t trust it completely. Someone – a human – has to control and fix AI output so it can meet quality expectations. Only then can the brand maintain trust and credibility with their customers.
MT, the earliest form of AI translation, has been around since the 1950s. For all that time, LSPs have been working on ways to ensure the output of AI translation meets quality standards, including how to involve humans in the post-edit of content after it has been translated by AI.
Monika: Yes, but things are changing now with LLM models because they are not trained exclusively on cleaned translation data like past MT models but rather on all data. Also, LLMs work on patterns rather than using rules or statistics like earlier AI translation models. We can’t predict the output of the LLM like we could with the former AI translation models. The errors are now actually different. Machine translations are more accurate when customized for a specific domain, but less fluent and creative. On the other hand, LLM translations are more fluent and creative but less accurate because they aren’t trained on a specific domain.
LLMs are a powerful tool that can supplement or even challenge traditional MT systems. We know how to train MT engines, customizing them with data, translation memory, glossaries, etc. Traditional MT is fully trainable, and the output follows rules. One way to ‘train’ an LLM is with prompts – providing any input, references, or instructions you think it needs to do a good job with your request. We’re figuring out, as fast as we can, how to leverage all the quality control processes – the language assets like glossaries and translation memories that have driven translation quality for years – into this new LLM model. It is then a matter of figuring out how to do the quality assurance at the end after the AI does the translation.
LLMs can also be “fine-tuned,” which is more like the traditional MT training. That requires time, money, and expertise, so we are seeing reliance on prompt engineering first.
So, what are some of the new ways we’re developing to manage quality with LLM?
Monika: It’s a lot about prompt engineering. Defining requirements and creating the best prompts will be a huge part of quality assurance. For example, there will be prompts around how to translate, what standards to use, what to use for references, and what samples to study for examples. Unlike traditional AI translation, LLMs can take additional input provided by humans at the time of translation.
We also need to rethink our approach to documents like style guides. AI can be trained, through prompts, on industry-specific terminology, writing styles, or even brand voice. We need to distill what is essential for the brand and how to prompt AI to capture a brand.
We also need to understand where we might see the bias and hallucinations and identify how we minimize the risk of those occurring, and then later, through a human post-editing process, how to remove those errors when they appear.
I foresee in the near future, there will be new roles on linguistic quality teams, such as translation prompt engineers. What might this look like?
Monika: Yes, I see that role emerging, and people will need to be trained to do this. It’s all new. Whoever does it must have a good understanding of content quality because creating excellent translations has a lot to do with how good the source content is. They must also understand how LLMs work and fully understand creating prompts, including how the output changes based on the order of information and what should be included or omitted in the prompt. This will need to be someone who has a lot of hands-on expertise working with LLMs, has a solid background in content creation, understands translation processes, and knows how to write prompts.
You mentioned the quality of source content. It’s a best practice in localization to optimize source content so it will be easy to localize and minimize translation errors. High-quality translations come from high-quality source content. So, do you think that optimizing the source for translation is more important now than ever?
Monika: We have all seen content that was not ready for localization, and what a mess that can cause. Any error missed at the source creation step will have a ripple effect, especially if you have many languages in your portfolio. So, one error becomes 70.
But in this age of ‘everything AI,’ when the source was also created by AI, you can’t assume that the source content was correct, high-quality, or optimized for localization to start with. You cannot only focus on the quality of the translated output.
The source will need to be reviewed before AI translation for the best results. In the source, for example, is the content free of bias? Is the material aligned with the company brand, tone, and feel? Also, where might hallucinations and bias occur when it’s translated? This review will involve changing the source and correcting any existing errors. And then we send it for AI translation, and all that review needs to happen again. That’s post-editing.
For most brands, it’s just too risky to have one person at the very end of that cycle who is responsible for the translation quality. So you need to have one pre-editing for quality at source and then another post-editing after translation. As the outputs improve, you can reevaluate your checks and balances, check less often for languages that perform well, and move your effort to language pairs that are underperforming. Even if the prompts are great, if you want top quality, there will always be steps where humans step in and evaluate the output, both at the original source and the translations.
So how do traditional methods of AI translation, like Neural Machine Translation, interplay with LLM models? Is there a synergy there? Each has different strengths and benefits.
Monika: Everyone in the industry is evaluating options to see where the most significant benefits are. There are a lot of data and assets like translation memories and glossaries that are part of the NMT model. These assets need to be leveraged to optimize the LLM model of translation. Currently, companies are figuring out how to integrate LLM with translation memory systems and traditional computer-assisted translation tools such as quality evaluation.
Top technologists are figuring out how to drive quality when using AI. But as of now, it’s risky for enterprises to just switch to AI content creation and AI translations without adjusting existing processes and adding safeguards because the impact of errors, bias, and hallucinations on a brand and on consumers is very high. Customers will lose trust in the brand if the content contains bias and errors. In contexts that require very accurate or concise/explicit instruction, such as in healthcare, legal, and government settings, errors can have devastating results.
As an industry, we are working out these problems right now. New roles will come of it. We must continue to stand by quality as a driver of consumer trust, understand the quality risks in the AI process, and proceed carefully. We have to identify the points where quality is at risk and insert humans to ensure the outcome meets our desired standards.
We can mitigate the risks. We’re better safe than sorry here. But being safe does not need to mean expensive.
We have to remember that we create content for humans. AI can, to some extent, tell you what might appeal to a specific target group. However, it’s a human who knows how to elicit particular emotions, create brand connections, and connect with people on a human level. After AI does its work, a human needs to look at the content and determine whether it’s high quality or if we need to fix it to meet our standards.
I think the beauty of our industry is that it keeps evolving, right?
When I started 20 years ago, Trados was the ‘wow thing’ in the market. It was new because many people were not using any CAT tools at all. Even with the introduction of machine translation, people predicted the end of the industry and the end of human linguists, and it didn’t happen. It wasn’t true then, and it won’t be true now.
It’s a roller coaster right now with all the advancements in AI translation. Not a high-speed train, but a roller coaster. It’s really exciting.
To ensure you are well-equipped to navigate the complexities of the evolving global marketplace, contact us today.
Takeaways from Monika on translation quality in the Age of AI
Language services are working hard to adapt existing translation quality processes and drive new ones to fit the new LLM translation models. No one will set previous AI translation models aside, but quality processes and best practices will change to fit the new generative models. Translation prompt engineering will be critical, and we will see jobs with this specific area of expertise in the language services industry. Tools integrations will also be crucial, with engineers figuring out how CAT tools like translation memory will work with LLMs. MT and LLM aggregation and integration will be a topic at industry conferences.
And we’re thrilled to be in the middle of it.
If you are using or want to use AI translation and are concerned about how you will control quality at source or after translation, our experts like Monika can guide you. Connect with a Vistatec expert here for a conversation.
As part of our Insights Unveiled series, each month we are releasing new perspectives and insights on the current and future landscape of the localization industry. Sign up below to ensure you don’t miss out on more insights, answers, and predictions about the future of localization.
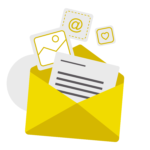
Sign up to this content series and be the first to be notified when new content is released
Sign up to this content series and be the first to be notified when new content is released
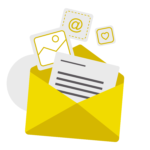